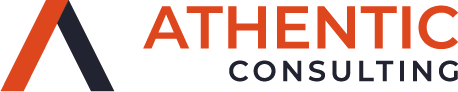
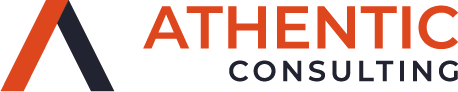
A data strategy is the overarching approach that defines how an organization will manage, govern, and utilize data to achieve its broader business objectives. With nearly 70% of digital transformation initiatives failing to meet expectations, a well-crafted data strategy serves as a critical enabler for success. This article outlines a proven three-phase approach to building an effective data strategy: Contextualization (understanding your organization's data landscape), Ideation (identifying high-value use cases), and Prescription (planning for execution). By following this framework, organizations could transform data from a byproduct of business operations into a driving force for innovation and growth.
Key Takeaways:
A data strategy is not merely another technical initiative or an isolated plan for using data analytics or AI. Rather, it represents the overarching strategy of an organization's entire data function - a function that serves internal data consumers across the enterprise. What distinguishes a true data strategy is that strategic decisions about data are deeply embedded within and supportive of the organization's broader business strategy.
The need for robust data strategies has grown exponentially over the past decade, paralleling the unprecedented growth in data generation and storage within organizations. This data explosion demands a well-defined, strategic approach to effectively manage information assets, enhance analytics capabilities, and leverage technology to unlock their full value. For more information, refer to our previous article: Answering 5 key questions about data strategy
The University of California, Berkeley provides a structured approach to developing a data strategy through a three-phase framework. While Berkeley established this conceptual structure, the following key activities within each phase reflect our practical experience implementing this framework in Thailand:
Goal: To develop a clear picture of the organization's current position, available resources, and how its data landscape aligns with strategic business goals.
Key Activities:
This phase establishes the critical foundation for all subsequent strategic data decisions by ensuring alignment with business objectives from the outset.
Goal: To identify tangible data use cases that directly address organizational pain points while supporting strategic objectives.
Key Activities:
When determining which use cases to ideate and prioritize, we apply Harvard's defensive/offensive framework at this stage. Each potential use case can be classified as either defensive (focused on protection, governance, and data quality) or offensive (focused on analytics, AI, and value creation). This classification ensures a balanced and strategically aligned portfolio of initiatives, reflecting the organization’s priorities identified during the Contextualization phase.
Additionally, the use cases typically fall into two categories:
We recommend identifying between one to five 'major' use cases, and one to three 'quick wins', depending on the size of the organization and the budget for these initial steps into strategic use of data.
Finally, the prioritization process should involve scoring each use case based on management input, strategic alignment, and implementation complexity and feasibility.
Goal: To develop detailed implementation plans for prioritized use cases, ensuring they can move from concept to execution.
Key Activities:
This phase transforms abstract concepts into concrete, actionable plans with clear ownership, timelines, and success metrics.
A data strategy serves as the essential blueprint that aligns an organization's data function with its broader objectives. In today's data-intensive environment, implementing a structured approach through the three phases—Contextualization, Ideation, and Prescription—provides a flexible framework that can be adapted to any organizational context.
The beauty of this three-phase approach lies in its adaptability. While the methodology remains consistent, the specific outcomes and priorities naturally differ based on organizational type. For example, private sector organizations may prioritize cost efficiency and profit generation, whereas public sector entities might emphasize service improvement, transparency, and alignment with policy or regulatory requirements.
Regardless of the sector, the fundamental value proposition remains the same: transforming data from a passive byproduct of operations into a strategic asset that drives mission fulfillment. The three-phase approach provides the structure, while the specific implementation adapts to the unique context and objectives of each organization.
A well-crafted data strategy is not a rigid template but a flexible framework that acknowledges the distinctive nature of each organization while providing a structured path to unlock the value of data assets in service of specific missions and goals.